What is Data Analytics?
Types of Data Analytics
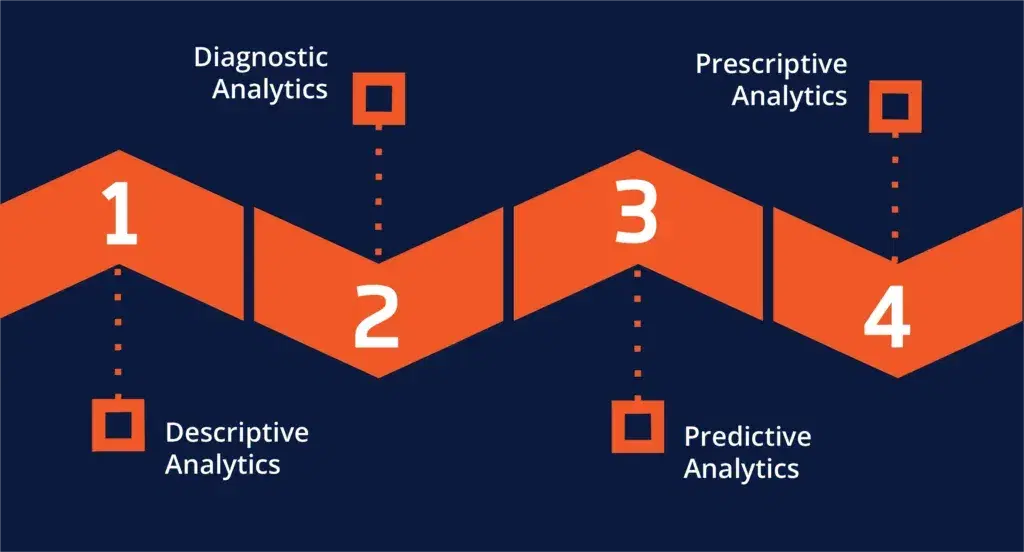
- Descriptive Analytics:
Descriptive analytics answers the question, “What happened?” It involves summarizing past data to understand trends and patterns. Examples include monthly sales reports, website traffic summaries, or patient admission statistics. - Diagnostic Analytics:
Diagnostic analytics focuses on understanding “Why did it happen?” It looks at data to find the causes of specific outcomes, using techniques like root cause analysis and correlation analysis. - Predictive Analytics:
Predictive analytics addresses “What is likely to happen?” It uses historical data, statistical models, and machine learning to forecast future outcomes, such as customer churn, demand fluctuations and patient readmission risks. - Prescriptive Analytics:
Prescriptive analytics answers “What should we do?” It provides recommendations or solutions to optimize decision-making. Examples include suggesting the best marketing strategies, resource allocation, or personalized treatment plans in healthcare.
Why Data Analytics is Becoming So Important?
How Data Analytics helps in healthcare?
Benefits Data Analytics Can Provide Your Healthcare:
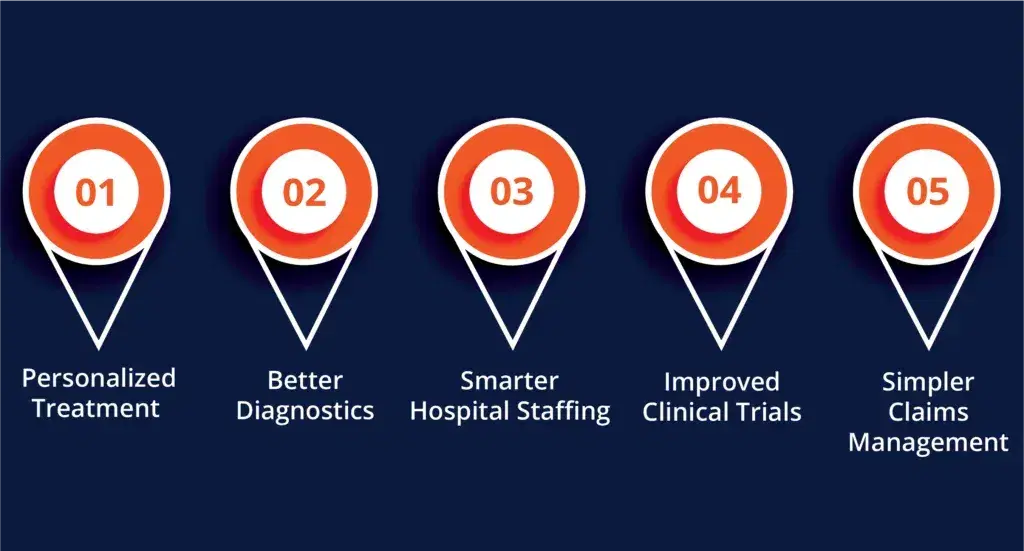
- Personalized Treatment:
By looking at a patient’s health records, history, and medication, data analytics helps doctors suggest the best treatments for each patient. This makes care more accurate and focused on the person. It also helps doctors adjust treatment plans based on the latest information, so patients always get the most suitable care as their condition changes. This leads to better health outcomes and more satisfied patients. - Better Diagnostics:
Data analytics, along with tools like artificial intelligence (AI), helps study medical images like X-rays or MRIs to find problems that doctors might miss. This makes diagnoses faster and more accurate. With the help of AI, these tools can improve over time, learning from new data. This helps doctors detect diseases earlier, giving patients a better chance of successful treatment and saving money by avoiding expensive late-stage treatments. - Smarter Hospital Staffing:
Data analytics helps predict how many staff members a hospital needs at different times. This helps hospitals avoid having too few or too many workers, making them more efficient. By looking at past data and trends, hospitals can predict when they’ll be busy and make sure there are enough doctors and nurses available. This way, they can keep costs low, reduce extra hours, and make sure patients always get the care they need. - Improved Clinical Trials:
In medical research, data analytics helps check test results to ensure that new medicines or vaccines are safe before being approved. It helps doctors and scientists see how well the treatments work, faster. By looking at large amounts of data, researchers can spot side effects or problems early. This helps speed up the approval process so new treatments can be used by patients sooner. It also helps make sure the right people are tested, which makes the results more reliable. - Simpler Claims Management:
Data analytics helps hospitals handle bills and insurance claims more easily. It reduces mistakes and ensures payments are made on time. By using data tools, hospitals can quickly spot problems with bills and fix them before they cause delays. This makes the claims process faster and more accurate. With better management of bills and insurance, hospitals can reduce costs and spend more time focusing on helping patients.
Data Analytics in Healthcare
Some of the Major Impacts of Data Analytics in Healthcare
Predictive analytics has a massive impact on reducing hospital readmission rates. By analyzing patient histories and identifying common characteristics of readmitted patients, hospitals can develop strategies to reduce these occurrences. For instance, hospitals use predictive models to identify patients at risk of readmission due to chronic conditions and offer proactive follow-up care. This not only reduces costs but also improves patient care by preventing avoidable re-hospitalizations.
Data analytics is also changing how new drugs are developed. Traditionally, drug development was a long and costly process. Now, with the help of advanced data analytics, pharmaceutical companies can speed up the discovery process by analyzing clinical trial data, predicting patient responses to treatments, and identifying new compounds with potential. This saves time and resources, making it easier to bring life-saving drugs to market faster.
Population health management uses data analytics to improve health outcomes for a specific group of people. By analyzing large datasets, healthcare providers can identify health trends within a population, such as high rates of diabetes or heart disease. With this information, they can create targeted interventions and wellness programs to improve the overall health of the population while reducing healthcare costs.
In busy places like emergency rooms (ERs), it’s really important to manage resources well to save lives. Data analytics can help by predicting how many patients will come in, based on things like the time of day, past trends, and even factors like weather or local events. By looking at past ER data, hospitals can plan staffing better, make sure the right number of doctors and nurses are available, and reduce wait times. Predictive models can also help spot patients who might get worse quickly, so they can be treated first. This makes care faster, reduces overcrowding, and helps doctors focus on the most serious cases.
Data analytics can also help healthcare leaders make better decisions that improve people’s health. By looking at data about diseases, population health, and how well current health programs are working, policymakers can spot areas that need more attention. For example, predictive analytics can help plan for the healthcare needs of an aging population or identify areas where more doctors, hospitals, or clinics are needed. With better data, healthcare policies can improve the way health services are delivered and make sure everyone has better access to care.
Get a Brighter Future in Healthcare with Better Data Analytics
Let’s Understand the Role of Data Analytics in Healthcare with A Real-Life Example
Scenario: Data Analytics for Predicting Patient Treatment
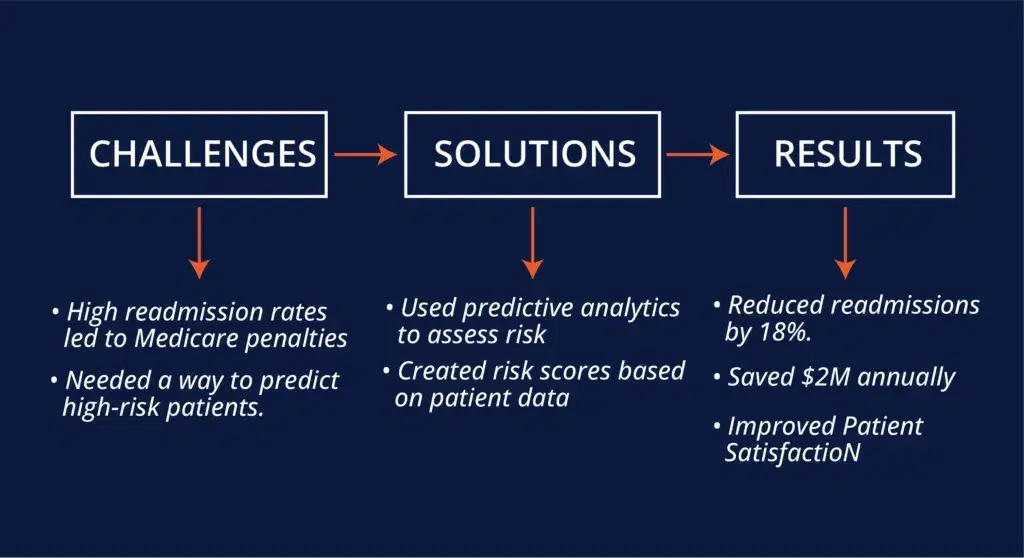
Predictive Analytics in Healthcare: Helping Doctors Save Lives
This kind of prediction is also useful for patients who are recovering from surgery. Data can help doctors figure out if a patient is at risk of getting sick again or needing to go back to the hospital. By doing this, doctors can take action earlier, helping patients recover better and faster. Hospitals can also use this data to plan how many doctors, nurses, and hospital beds they need. If they know more patients will come in at certain times, they can be better prepared to help everyone without making them wait too long.
Final Thoughts
As highlighted in the example of the Chicago healthcare network, utilizing predictive analytics not only reduced readmission rates but also delivered significant financial and patient care benefits. This real-life scenario underscores the potential of data analytics to address pressing challenges in healthcare while paving the way for a brighter and more efficient future.