Introduction
In this tutorial we explore how we can connect OpenAI’s Chat Completions API to external services through function calling. This capability allows the model to generate JSON objects that can serve as instructions to call external functions based on user inputs.
Understanding Function Calling in the Chat Completions API
The Chat completion API provides us with an optional parameter “tools” that we can use to provide function definitions. The LLM will then select function arguments which match the provided function specifications.
Common Use Cases
The completion response is computed and after that it is returned.
get_current_location()
send_friend_request(userId) ·
2. Connect to SQL Database: Using Natural language, define functions that can make request to database queries.
How It Works
Integrating LLMs with external tools involves a few straightforward steps:
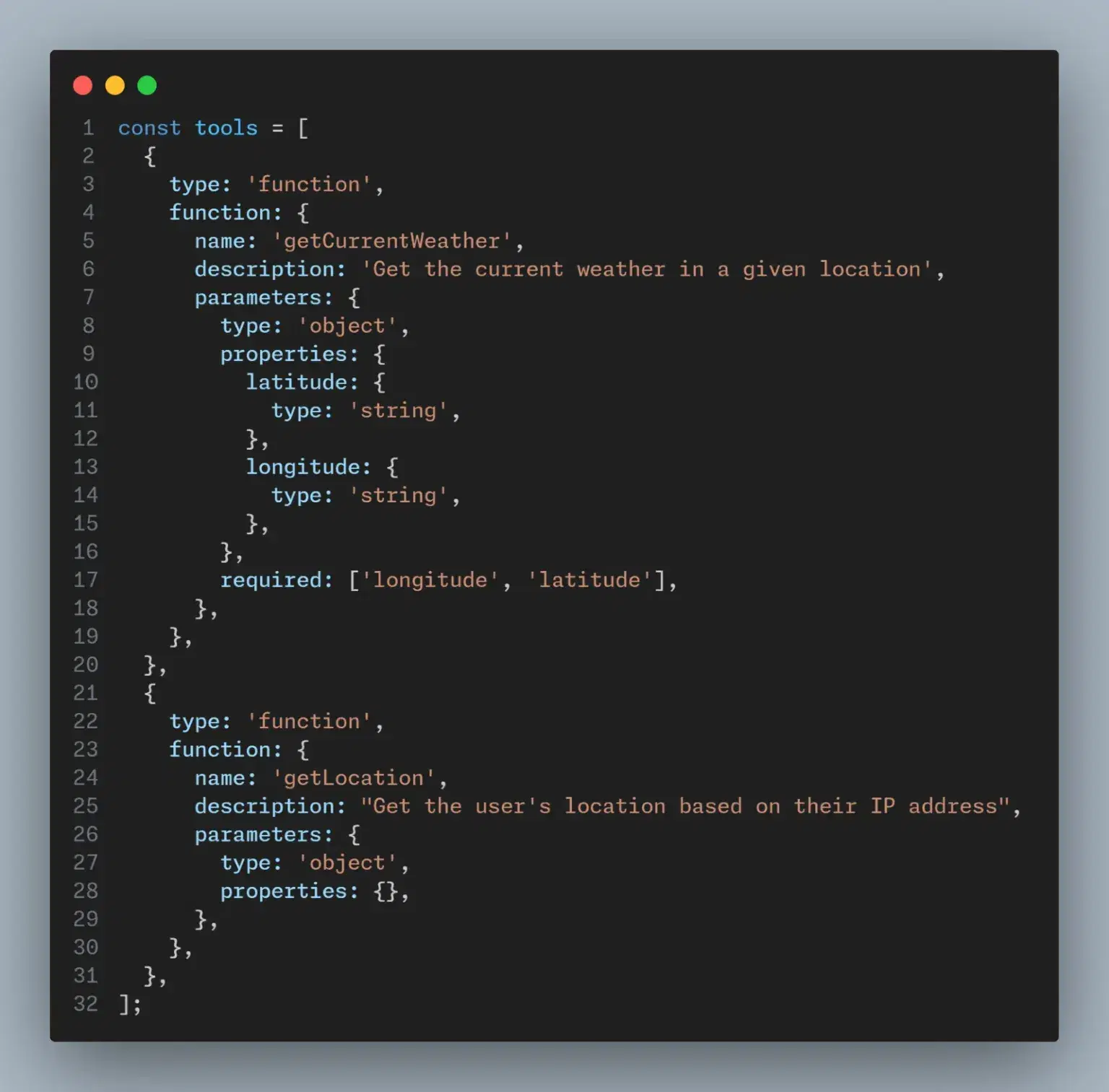
2. Call the Model: Send the user’s query to the model. The model processes the input and determines whether and which function to call, returning a JSON object that represents the function call.
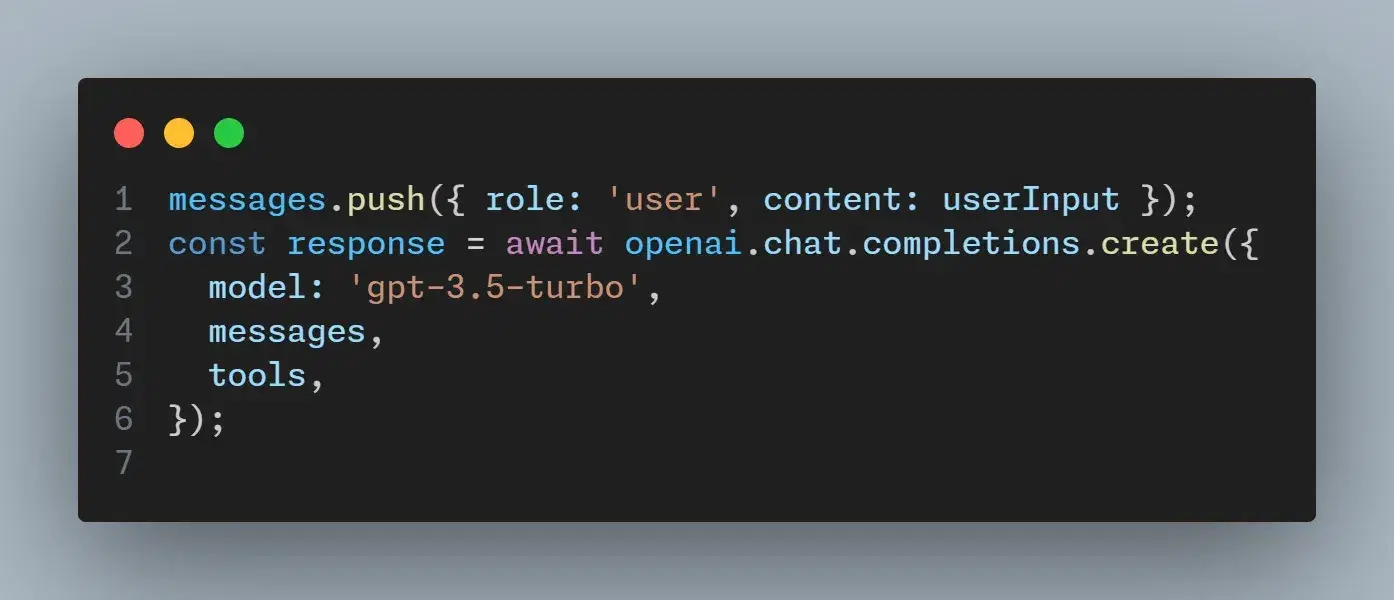
3. Handle the Output: The JSON output from the model will contain the necessary details to call the external function. This may include function names and argument values.
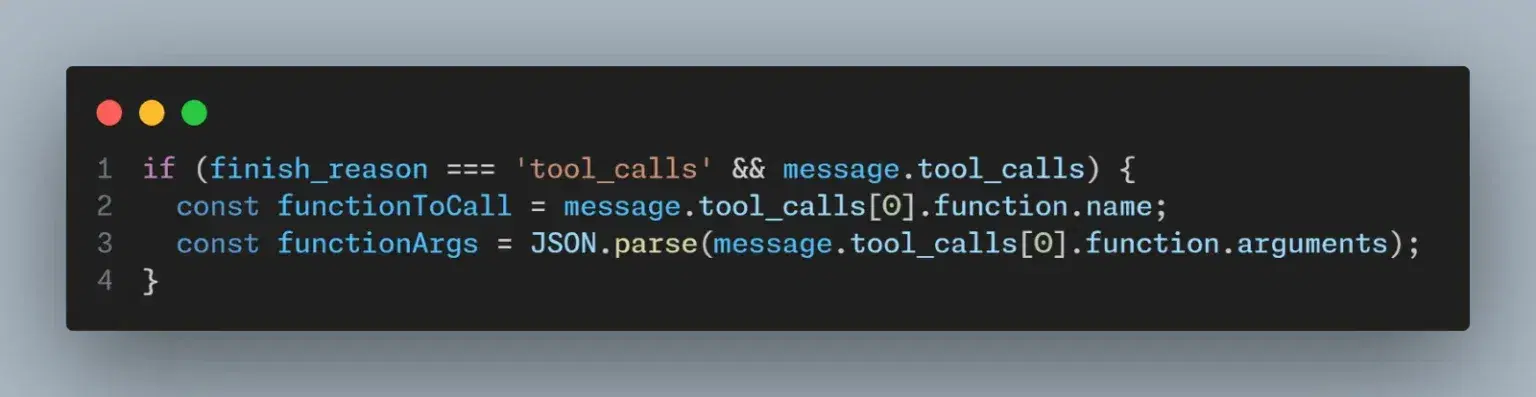
If a tool was called, the “finish_reason“ in the ocmpletion respnse will be equal to “tool_calls“. Also, there will be a “tool_calls” object that will contain the name of tool as well as any arguments.

5. Process and Respond: After executing the function, you might need to call the model again with the results to generate a user-friendly summary or further instructions.

Conclusion
Integrating OpenAI’s Chat Completions API with external tools improves the functional capabilities of applications. By defining functions and utilizing the ‘tool’ parameter, developers can create interactions that use real-time data and actions to increase the responsiveness and versatility of their software.