Introduction:
Why Databricks Performance Matters
1. Leverage Delta Lake for Reliable and Faster Data Processing
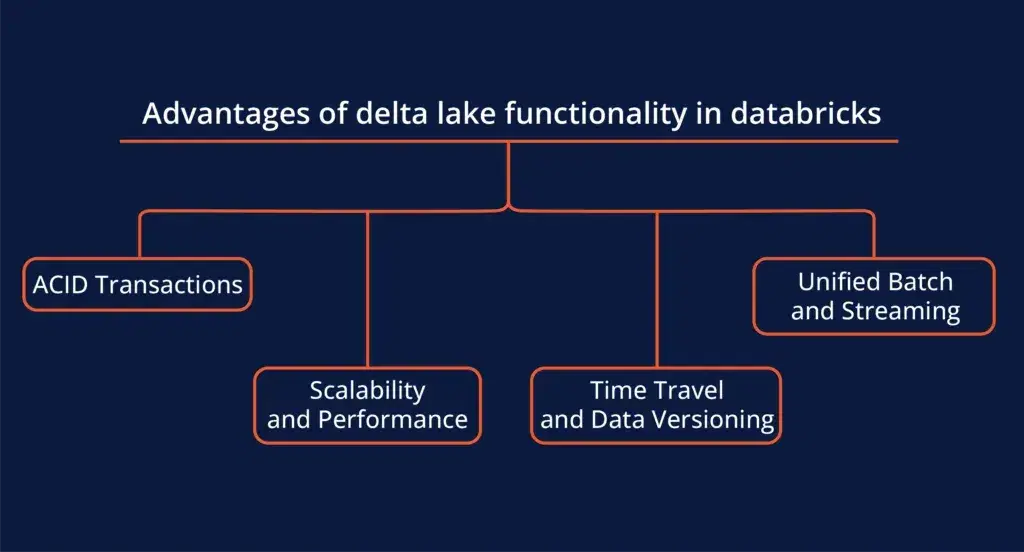
- ACID Transactions: Delta Lake offers ACID (Atomicity, Consistency, Isolation, Durability) transactions, meaning your data stays reliable even during simultaneous reads and writes.
- Version Control: Delta Lake’s versioning capabilities allow you to track changes, roll back to previous versions, and recover corrupted data instantly.
2. Maximize Resource Utilization with Auto-Scaling Clusters
- Automated Scaling: Whether you’re running an intense machine learning model or processing large datasets, auto-scaling adjusts the cluster size to meet real-time needs without manual intervention.
- Reduce Idle Time: By automatically terminating idle clusters, you can avoid paying for unused resources, which is particularly useful if you’re running AWS Databricks or Azure Databricks in a cost-conscious environment.
3. Efficient Machine Learning with Databricks AI
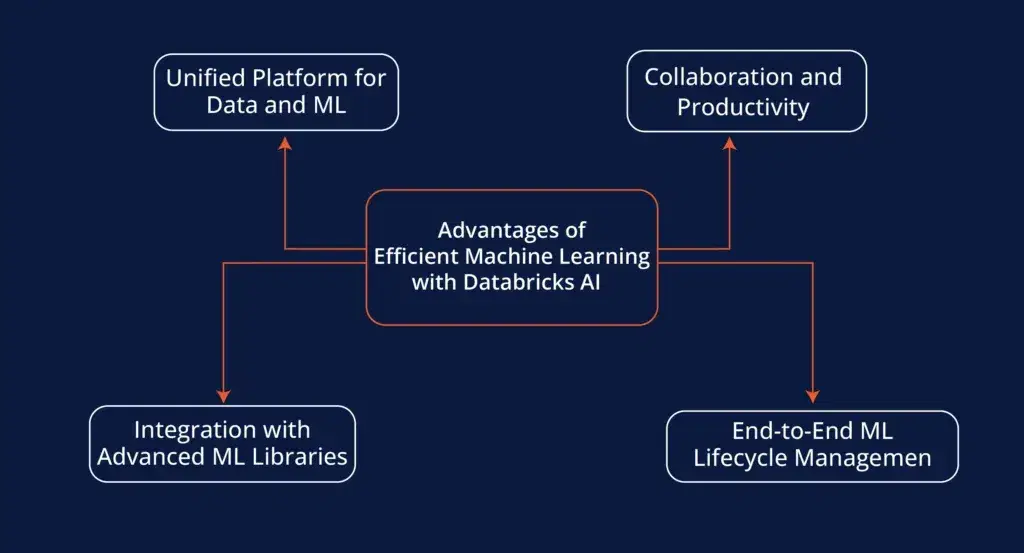
- Seamless Model Training: Databricks AI allows you to train machine learning models directly on your live data, giving you real-time predictions and insights.
- Integration with AutoML: If you’re new to machine learning, Databricks AI also supports AutoML (Automated Machine Learning), helping you build robust models without deep AI expertise.
4. Improve Query Speed with Caching
- DataFrame Caching: By caching DataFrames, you eliminate the need to recompute results, making your pipeline significantly faster.
- Selective Caching: Not all data should be cached. Focus on frequently used data to avoid wasting memory on rarely accessed information.
5. Automate Workflows with the Databricks API
- Custom Automation: Use the API to create custom workflows that automatically execute at certain intervals, freeing up time for more important tasks.
- Integration with Other Tools: The Databricks API can easily be integrated into your existing tools or CI/CD pipelines, allowing you to automate data engineering tasks end-to-end.
6. Collaborate Across Teams Seamlessly
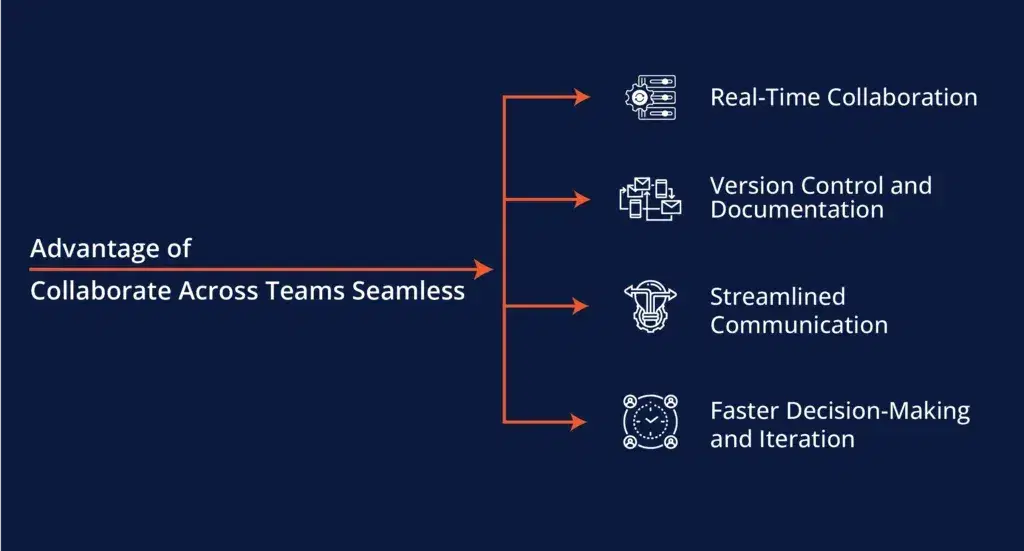
- Real-Time Collaboration: Multiple team members can work on the same notebooks and pipelines simultaneously, making collaboration smooth and error-free.
- Version Control: Keep track of all changes and easily roll back to previous versions if needed. This is a big deal for teams working on complex data models and transformations.
7. Databricks vs Snowflake: Performance and Efficiency
- For Advanced Analytics: If your focus is on data engineering, machine learning, or real-time data analytics, Databricks is the superior choice.
- For Data Warehousing: If you need a straightforward data warehousing solution without AI capabilities, Snowflake may be more cost-effective.
8. Monitor Performance with Built-In Tools
- Cluster Monitoring: Track CPU and memory usage in real-time to ensure that your clusters are running efficiently.
- Job Monitoring: Keep tabs on job execution and set up automated alerts to notify you of any failures or performance drops.